Workshop on Scientific Methods for Understanding Deep Learning
Workshop at the Conference on Neural Information Processing Systems (NeurIPS) 2024
Accepted papers: OpenReview.
Videos of talks and panel session: NeurIPS website.
The workshop was held on Sunday, December 15th in West meeting rooms 205-207.
For latest news about the workshop, follow @scifordl on X/Twitter.
Keynote Speakers
Panelists
Organizers
Questions?
Contact us at scienceofdl.workshop.2024@gmail.com or @scifordl.Sponsors
We thank the Simons Foundation for their generous financial support.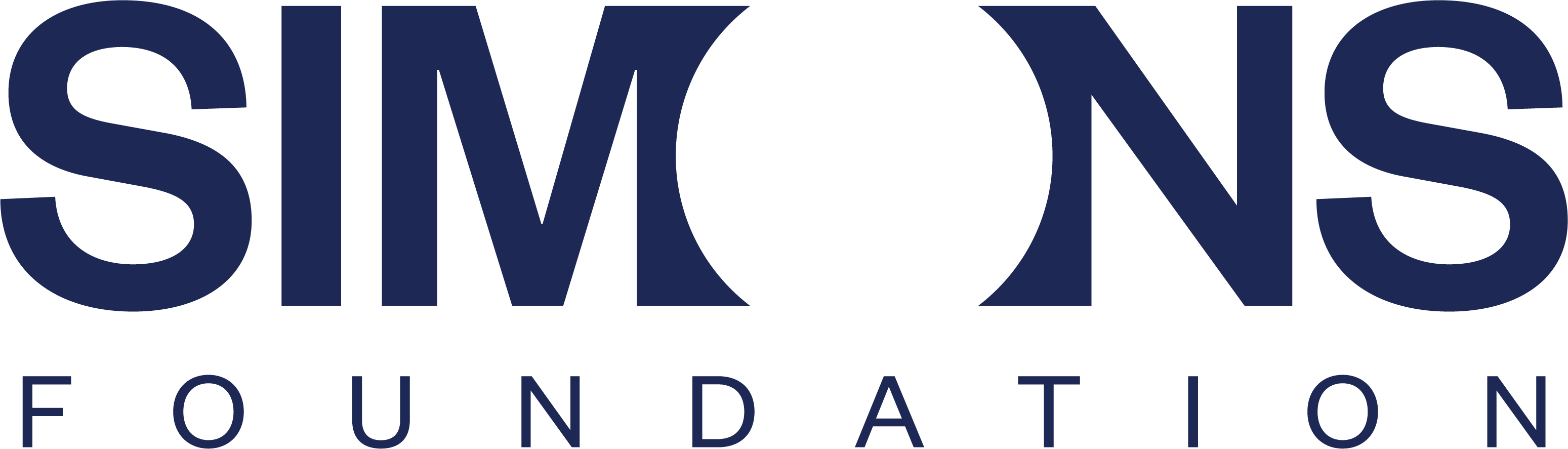